AI models may aid intrahepatic cholestasis of pregnancy prediction
Models able to distinguish healthy women from mild, severe ICP cases
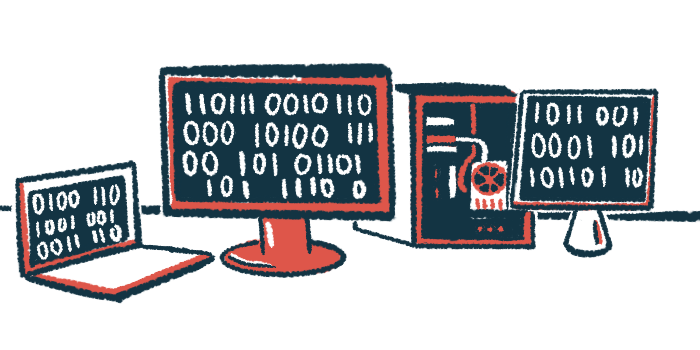
New artificial intelligence (AI) models based on 11 possible risk factors of intrahepatic cholestasis of pregnancy (ICP) can accurately distinguish between healthy pregnant women, those with mild IPC, and those with severe ICP, a study shows.
While the predictive potential of these models must be confirmed in larger studies, the findings suggest the models may be used in a clinical setting to help diagnose and grade the severity of ICP, improving care for both mothers and their babies.
The study, “Risk factors and machine learning prediction models for intrahepatic cholestasis of pregnancy,” was published in BMC Pregnancy and Childbirth.
ICP is a form of cholestasis that usually develops in the second or third trimester. In cholestasis, flow through the bile ducts, which take bile from the liver to the intestine to help with digestion, is slowed or stopped. As a result, bile acids build up in the liver and leak into the bloodstream, causing symptoms such as pruritus (itching). Its diagnosis is mainly based on the presence of pruritus and high blood levels of bile acids, the main component of bile, in pregnant women. This approach may delay or miss a diagnosis, however.
Given that ICP can increase the risk of complications for both mothers and babies, identifying risk factors early could help doctors keep mothers and their babies safe.
Separating healthy pregnancies, cases of ICP
Researchers in China turned to machine learning to diagnose ICP and determine how severe it is. Machine learning is a form of AI that looks for patterns in large amounts of data and applies lessons learned to make decisions or predict outcomes.
“Machine learning models are highly promising predictive tools and have been widely applied in clinical diagnosis and treatment over the years,” wrote the researchers, who noted “a relative paucity of studies focused on ICP.”
The researchers retrospectively analyzed data from 798 pregnant women seen at a Chinese hospital and divided into three groups: 300 with normal pregnancies (37.6%), 312 with mild ICP (39.1%), and 186 with severe ICP (23.3%).
High blood pressure during pregnancy, a known risk factor for severe ICP, was rare, suggesting little connection to disease severity.
By analyzing the data, the researchers identified 11 factors that appeared to play a role in predicting ICP and identifying its severity, including multiple pregnancy (twins or more), blood levels of total bile acids, and blood levels of the liver enzymes gamma-glutamyl transferase and aspartate aminotransferase.
Other risk factors were related to blood cells, including the number or percentage of lymphocytes, neutrophils, red blood cells, and platelets in the blood, the hematocrit, which is a measure of how much of the blood’s volume is occupied by red blood cells, and prothrombin time, a measure of how quickly blood clots.
Using data from these risk factors, the researchers tested 13 machine learning models. The five showing the greatest diagnostic potential were selected to optimize and evaluate.
One model, called CatBoost, “exhibited the best performance on both the training and validation [groups of patients],” and was able to discriminate between pregnant women with and without ICP with an accuracy of 90.85%.
The five models were better at correctly identifying healthy pregnant women and those with mild ICP than those with severe ICP, “possibly due to feature overlap” between the mild and severe ICP groups, the researchers wrote.
“These models demonstrated good performance and can be used to help predict whether pregnant women have ICP and the degree of ICP (mild or severe),” wrote the researchers, who noted that “studies with larger datasets are warranted to further validate and refine these predictive models.”
“By integrating machine learning techniques with readily available clinical and laboratory data, practitioners can more effectively identify women at risk for ICP. This enables more accurate counseling and management strategies, ultimately improving patient outcomes and ensuring timely intervention,” they wrote.