AI model may predict cholestasis-related cognitive impairment risk
Advanced age, elevated D-dimer levels associated with increased risk
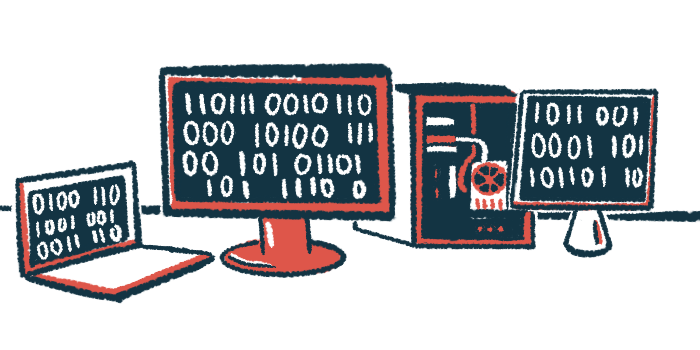
An artificial intelligence (AI) model can accurately discriminate between cholestasis patients with and without moderate to severe disease-associated cognitive impairment based on 13 possible risk factors, a study shows.
The model “demonstrated strong predictive performance and clinical utility, providing a robust tool for early identification of patients at risk of moderate to severe cognitive impairment,” which can subsequently result in early intervention, the researchers wrote. The study, “Leveraging machine learning for precision medicine: a predictive model for cognitive impairment in cholestasis patients,” was published in BMC Gastroenterology.
In cholestasis, the flow of the digestive fluid bile from the liver to the intestines is slowed or blocked, causing bile acids, the main components of bile, to build up to toxic levels in the liver and leak into the bloodstream. This leads to symptoms such as itching and yellowing of the skin and eyes, called jaundice.
“Emerging research highlights the deleterious impact of cholestasis on cognitive function, particularly in domains such as memory, attention, and executive functioning,” the researchers wrote. Executive function refers to the set of mental skills needed to plan, solve problems, and adapt to new situations.
What causes the neurological effects of cholestasis appears to involve several factors, including bodywide inflammation, a type of cellular damage called oxidative stress, nutritional deficiencies, and dysregulation between the gut, liver, and brain. Still, “cognitive dysfunction in cholestasis patients often remains underdiagnosed and poorly understood in clinical practice, necessitating robust predictive models to facilitate early identification and intervention,” wrote a team of researchers in China.
Using AI to augur cognitive impairment risk
In their study, the researchers turned to machine learning to predict the risk of moderate to severe cognitive impairment with cholestasis. Machine learning is a type of AI that looks for patterns in large amounts of data and applies them toward decision making or predicting outcomes.
The researchers retrospectively analyzed clinical and biochemical data from 353 cholestasis patients hospitalized at Qingyang People’s Hospital in China between 2021 and 2023. The patients were divided into those with moderate to severe cognitive impairment, defined as a Montreal Cognitive Assessment (MoCA) score of 17 or lower (16.1%), and those without significant impairment, or a MoCA score higher than 17 (83.9%).
Statistical analyses identified 13 potential risk factors that provided the best predictive accuracy for the model. These included older age, lower height, high levels of fasting blood glucose and D-dimer, low blood levels of total protein and albumin, and high platelet counts. D-dimer is a marker of blood clotting and inflammation, while low total protein and albumin indicate metabolic and liver problems. Platelets are the blood cell fragments involved in blood clotting, which can be affected by cholestasis.
“These findings underscore the multifactorial nature of cognitive impairment in cholestasis, highlighting the interplay between metabolic, inflammatory, and [blood clotting-related] pathways,” the researchers wrote.
Based on the 13 factors, the researchers tested 12 machine learning models for their ability to distinguish between those with and without moderate to severe cognitive impairment. A model called LightGBM showed the best performance and was able to discriminate between those with and without cognitive impairment with an accuracy of 79.6%.
“Considering both training and testing results, LightGBM strikes the best balance between high discriminatory ability and robust generalization,” wrote the researchers, who said further analyses identified advanced age and elevated blood D-dimer levels as “the most influential predictors” of increased risk of cognitive impairment
“The developed LightGBM-based model effectively predicts cognitive impairment in cholestasis patients, providing actionable insights for early intervention,” they wrote. “Integrating this tool into clinical workflows can enhance precision medicine and improve outcomes in this high-risk population.”
The researchers said studies in multicenter and diverse patient populations are needed to confirm the model’s predictive potential in all cholestasis patients and “incorporating additional biomarkers, such as inflammatory markers or neuroimaging, could enhance predictive accuracy.”