New AI model helps make accurate diagnoses of biliary atresia
Model's diagnostic potential outperformed less experienced specialists
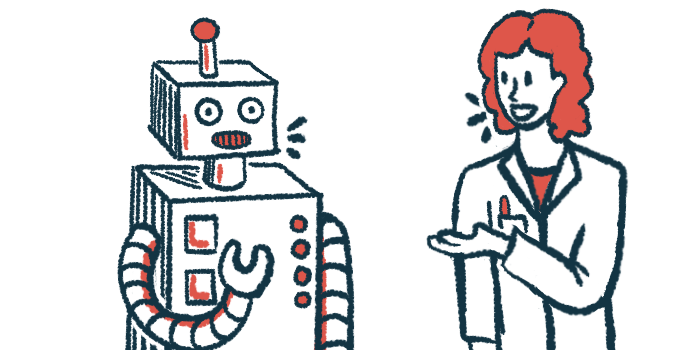
A new artificial intelligence (AI) model based on clinical data along with laboratory results and ultrasound images aids the early diagnosis of biliary atresia, a study shows.
The diagnostic potential of the model matched that of experienced experts and outperformed less experienced specialists, whose diagnostic performance improved by using it.
“The model’s high accuracy and its ability to enhance the diagnostic performance of human experts underscore its potential for significant clinical impact,” the study’s researchers wrote. The study, “Development of an artificial intelligence-based multimodal diagnostic system for early detection of biliary atresia,” was published in BMC Medicine.
Biliary atresia is a rare, but serious liver disease that affects infants. It’s marked by the absence or blockage of bile ducts, the tubes that transport the digestive fluid bile to the intestines. As a result, bile builds up in the liver to toxic levels, leading to damage and bile leakage into the bloodstream, which causes symptoms such as yellowing skin and whites of the eyes, called jaundice.
The first-line treatment is Kasai surgery, which is intended to restore bile flow by creating an alternate route to the intestine. The procedure’s best results are achieved when performed before 30 to 45 days of age, but, unfortunately, the “median age at the time of the Kasai procedure is approximately 60 days,” the researchers wrote. “The major cause for delay is the absence of effective and practical screening methods, thus presenting early diagnosis as a prominent and persisting clinical challenge.”
Using AI toward biliary atresia diagnoses
Researchers in China sought to address this challenge by applying deep machine learning, a form of AI that uses algorithms to learn from data and identify and predict patterns. The study’s first part, which was meant to train, validate, and internally test the AI model, included infants younger than 6 months with suspected biliary atresia or elevated blood levels of bilirubin, a marker of liver damage, along with those without liver disease.
There was a total of 681 biliary atresia patients and 898 non-biliary atresia patients, who didn’t have liver disease or had other diseases marked by cholestasis, or stalled bile flow. The study’s second part, to test the model, included additional infants who met the same criteria as in the first part.
Analyzed data included demographic characteristics, medical histories, laboratory test results, and ultrasound images of the gallbladder, which is the organ where bile is stored, and the triangular cord sign (TCS), a marker for biliary atresia.
The model’s diagnostic performance was compared with that of four radiologists with variable years of experience in diagnosing biliary atresia (from 0 to more than 10 years). The diagnostic accuracy was reported as AUC values where a value of 0 means a perfectly inaccurate test and a value of 1 reflects a perfectly accurate test.
On the first part’s internal test, the AI model achieved an AUC of 0.99, outperforming all four radiologists, including the one with more than 10 years of experience, who achieved an AUC of 0.95. In the external test, the model achieved an AUC of 0.97, which wasn’t significantly different from that of the most experienced radiologist.
Incorrect diagnoses
There were six cases where the AI model failed to correctly diagnose biliary atresia. All had gallbladder data that was harder to interpret, suggesting its “performance may be influenced by the quality of the imaging data or by atypical presentations of the condition,” the researchers wrote.
The model also incorrectly diagnosed biliary atresia in five infants without the condition. Three of them were under a month old and the other two had choledochal cysts, a congenital anomaly of the bile ducts. All but one also had elevated blood levels of GGT, a marker for liver and bile duct damage.
“This indicates that the model may be more prone to false positives in very young infants and patients with choledochal cysts, as well as those with elevated GGT levels,” the researchers wrote.
When they used the AI to assist their diagnosis, the radiologists, especially those with less experience, improved their performance. In the external test, the AUC of the radiologist without experience rose from 0.67 to 0.90, while the radiologist with more than 10 years experience showed no improvement with AI assistance.
Using ultrasound and clinical information alone (excluding blood tests, which are more invasive), the model achieved an AUC of 0.98, similar to the model using all the data. Moreover, an AUC of 0.96 was achieved with imaging data from the gallbladder and the TCS alone, and when using clinical information and laboratory tests alone.
“These findings imply that [AI] demonstrates exceptional proficiency in detecting extremely subtle structural changes and managing intricate numerical tasks, which pose challenges for human interpretation,” the researchers wrote. “By integrating ultrasound images, clinical data, and laboratory results, our multimodal deep learning models achieved high accuracy, outperforming human experts in retrospective evaluations and demonstrating robust performance in prospective validation.”
The researchers said “a multicenter study involving diverse healthcare settings and patient populations is warranted” to confirm their findings’ “broader applicability.”